Research Pillar: Economics/Law and AI
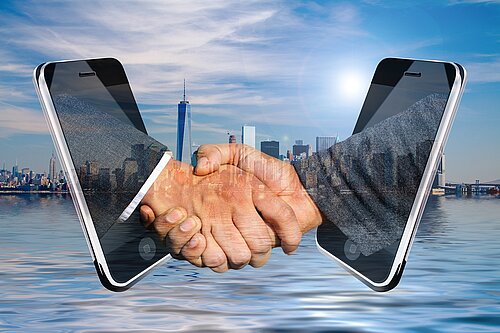
The research pillar "Economics/Law and AI" encompasses a diverse range of research areas that aim to understand and harness the potential of AI in various contexts. The focus of this pillar is on the practical application of AI to solve real-world problems, with a strong emphasis on interdisciplinary collaboration. The research areas within this pillar cover a wide spectrum of topics, including AI adoption in organizations, smart cities and urban mobility, smart industry and logistics, future energy systems, fraud detection and AI in law. One of the main goals of this pillar is to understand the challenges and opportunities that arise from the integration of AI in different domains.
Furthermore, the pillar also has a strong emphasis on the intersection of AI and law, with a specific focus on the legal challenges and opportunities arising from the use of AI. This includes research on the ethical and legal implications of AI, as well as the development of legal frameworks to support the safe and responsible deployment of AI.
Overall, the research pillar "Economics/Law and AI" aims to provide a comprehensive understanding of the impact of AI on society and to develop practical solutions that can be applied in the real world. Through interdisciplinary collaboration and a focus on practical applications, this pillar aims to contribute to the responsible and sustainable development of AI.
Research Areas
While there has been considerable progress in AI research, the adoption of AI in organizations is still lagging behind. This research area focuses on introducing AI in the practice of organizations. Thereby, AI is treated from a holistic perspective of technological, organizational and managerial capabilities that are required for a successful adoption in organizations.
Principal Investigators:
Publications:
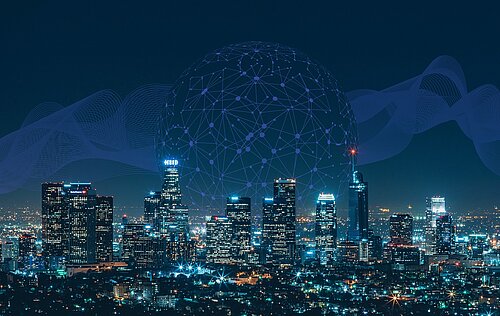
In the area of smart cities, we utilize urban data science to improve the quality of life of inhabitants and to make cities more efficient and sustainable. One key aspect of this is urban mobility, where we use AI to facilitate the integration of novel, more sustainable transportation modes, such as carsharing, shared bikes and e-scooters. This includes solving operational challenges as well as creating new business value potentials. Additionally, we also apply urban data science to other areas of smart cities, such as building energy management, waste management, and public safety. Overall, our goal is to use urban data science to make cities more livable, efficient, and sustainable for all inhabitants.
Principal Investigators:
Publications:
- 3D-PV-Locator: Large-scale detection of rooftop-mounted photovoltaic systems in 3D.
Applied Energy, 310:118469, 2022.
Kevin Mayer, Benjamin Rausch, Marie-Louise Arlt, Gunther Gust, Zhecheng Wang, Dirk Neumann and Ram Rajagopal.
[doi] [BibTeX]
- Predicting residential electricity consumption using aerial and street view images.
Applied Energy, 301:117407, 2021.
Markus Rosenfelder, Moritz Wussow, Gunther Gust, Roger Cremades and Dirk Neumann.
[doi] [BibTeX]
- The Constrained Reliable Shortest Path Problem in Stochastic Time-Dependent Networks.
Operations Research, 69(3):709-726, 2021.
Matthias Ruß, Gunther Gust and Dirk Neumann.
[doi] [BibTeX]
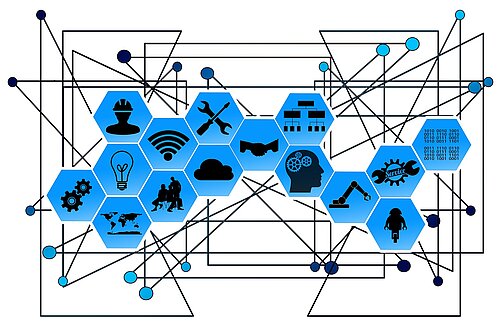
CAIDAS smart industry research focuses on utilizing AI to enhance the performance and efficiency of smart industry and logistics operations. This includes research and development in the areas of predictive maintenance, logistics optimization, and supply chain management, as well as the use of data-driven approaches for operations management. Additionally, the center explores the potential of reinforcement learning in these fields, for example, for autonomous decision-making in logistics and warehouse operations.
Principal Investigators:
Publications:
- Predictive End-to-End Enterprise Process Network Monitoring.
Business &$ Information Systems Engineering, 65(1):49-64, 2022.
Felix Oberdorf, Myriam Schaschek, Sven Weinzierl, Nikolai Stein, Martin Matzner and Christoph M. Flath.
[doi] [BibTeX]
- Catch me if you scan: Data-driven prescriptive modeling for smart store environments.
European Journal of Operational Research, 294(3):860-873, 2021.
Matthias Hauser, Christoph M. Flath and Frédéric Thiesse.
[doi] [BibTeX]
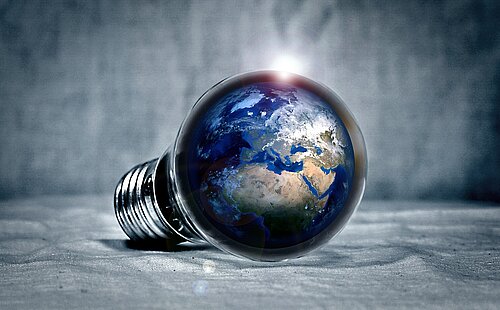
AI and data science have the potential to transform future energy systems by increasing efficiency, lowering prices, and enabling the integration of renewable energy sources. By forecasting and optimizing energy demand and supply, AI-based systems can be utilized to improve the performance of the power grid. Similarly, data science techniques can be used to find patterns and anomalies in massive collections of energy consumption data, allowing the development of more efficient energy management systems.
Principial Investigators:
Publications:
- Strategies for microgrid operation under real-world conditions.
European Journal of Operational Research, 292(1):339-352, 2021.
Gunther Gust, Tobias Brandt, Salman Mashayekh, Miguel Heleno, Nicholas DeForest, Michael Stadler and Dirk Neumann.
[doi] [BibTeX]
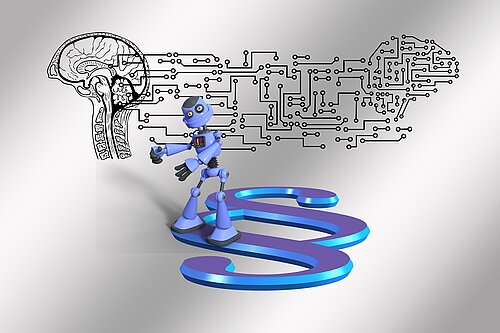
The area of "AI in Law" deals with the legal challenges posed by technological developments in the field of autonomous systems such as driverless motor vehicles, industrial or household robots, and new forms of artificial intelligence software. The Forschungsstelle RobotRecht (Robotics Law Research Centre) focuses on addressing liability law, criminal law, and data protection issues in a pragmatic way through cooperation with manufacturers, suppliers, users, and researchers. Another project is TargetJura, which aims to create an intelligent, case-based learning environment, where the resulting tutor system will be able to recognize argumentation structures independently. The AI will be used both in providing feedback for legal solutions and in stimulating discussions on ethical issues of AI through concrete case examples. The chatbot will ensure that the discussions are conducted according to certain rules and will address important arguments in a natural form without expressing a certain opinion.
Projects:
Principal Investigators:
Publications:
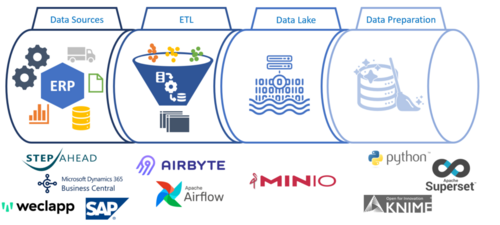
Fraud detection involves the development of methods for the reliable detection of fraud, for example in ERP systems or financial transaction data. The focus is on anomaly detection approaches and their adaptation for fraud detection, for example through the efficient modeling of numerical data dependencies or the explanation of model decisions through methods of explainable AI.
Projects:
Principal Investigators:
Publications:
- Open ERP System Data For Occupational Fraud Detection.
2022. cite arxiv:2206.04460.
Julian Tritscher, Fabian Gwinner, Daniel Schlör, Anna Krause and Andreas Hotho.
[doi] [abstract] [BibTeX]
- Financial Fraud Detection with Improved Neural Arithmetic Logic Units.
Lecture Notes in Computer Science book series (LNCS, volume 12591). volume Fifth Workshop on MIning DAta for financial applicationS. number 5. Springer, 2020.
Daniel Schlör, Markus Ring, Anna Krause, and Andreas Hotho.
[abstract] [BibTeX]
- iNALU: Improved Neural Arithmetic Logic Unit.
Frontiers in Artificial Intelligence, 3:71, 2020.
Daniel Schlör, Markus Ring and Andreas Hotho.
[doi] [abstract] [BibTeX]
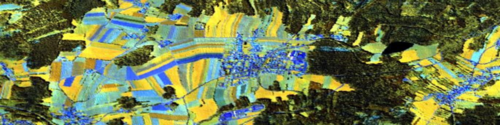
Remote sensing is a method of collecting and analyzing data about the Earth's surface and atmosphere. These data provide valuable information for a wide range of fields including demographics, economics, politics, culture, and environmental science.
In the last decade, there has been tremendous development in remote sensing: More and more digital imagery data, acquired by active and passive sensors on Earth-observing satellites, aircraft, and drones, is available. The sensors measure the reflected and emitted radiation from objects on the Earth's surface and in the atmosphere in various electromagnetic wavebands and different spatial scales.
But it is not just the variety and quantity of data that is considered a game changer in remote sensing. The rapidly increasing capacities for storage and processing and also new methodological possibilities in the field of machine learning and artificial intelligence make it possible to work on a larger scale or even globally. Deep learning methods, as an example, are being used very successfully to detect objects on the Earth's surface and describe their properties, localize them in space, and track changes over time. Thematic application fields are then, for example, the analysis of urban growth, the greening of cities, the exposure to natural hazards such as floods, or air pollution.
Principal Investigator:
Publications:
- Understanding an urbanizing planet: Strategic directions for remote sensing.
Remote Sensing of Environment, 228:164 - 182, 2019.
Zhe Zhu, Yuyu Zhou, Karen C. Seto, Eleanor C. Stokes, Chengbin Deng, Steward T.A. Pickett and Hannes Taubenböck.
[doi] [abstract] [BibTeX]
- The morphology of the Arrival City - A global categorization based on literature surveys and remotely sensed data.
Applied Geography, 92:150-167, 2018.
H. Taubenböck, N.J. Kraff and M. Wurm.
[doi] [BibTeX]
- Measuring morphological polycentricity - A comparative analysis of urban mass concentrations using remote sensing data.
Computers, Environment and Urban Systems, 64:42-56, 2017.
H. Taubenböck, I. Standfuß, M. Wurm, A. Krehl and S. Siedentop.
[doi] [BibTeX] - 3D-PV-Locator: Large-scale detection of rooftop-mounted photovoltaic systems in 3D.
Applied Energy, 310:118469, 2022.
Kevin Mayer, Benjamin Rausch, Marie-Louise Arlt, Gunther Gust, Zhecheng Wang, Dirk Neumann and Ram Rajagopal.
[doi] [BibTeX]
Principial Investigators
Gunther Gust
Business Informatics & Artificial
Intelligence in the Company
Christoph Flath
Information Management
Frédéric Thiesse
Business Informatics and Systems Development
Carlo D'Eramo
Reinforcement Learning and Computational Decision Making
Hannes Taubenböck
Global Urbanization and
Remote Sensing
Eric Hilgendorf
Information and Computer Science Law
Frank Puppe
Artificial Intelligence and Knowledge Systems
Andreas Hotho
Data Science